Beyond Automation: How GenAI Informs Next Best Action in P/C Insurance Claims and Underwriting
April 22, 2025
By Stan Smith
The insurance industry is increasingly adopting AI to streamline operations, automate tasks and improve customer experiences. But while AI automation, in particular, has become a standard assistive tool in many insurance professionals’ tool stacks, it has significant limitations—especially in guiding greener professionals through complex claims and underwriting situations.
Generative AI (GenAI)—the latest AI technology that generates data-driven responses and ideas—represents a novel opportunity to support these less-experienced insurers and even seasoned veterans with reliable recommendations for next steps. For example, GenAI models can analyze customer interactions in real time and recommend policy upgrades, risk management strategies or proactive measures to avoid costly claims and losses. In these scenarios, GenAI lifts some of the burden off understaffed and overworked insurance companies, giving employees the tools and data-driven recommendations they need to determine the next best action.
GenAI’s Role: Next Best Action in Smart Claims Management
Insurance claims processing historically has been an intricate and nuanced process, requiring junior staff and experienced adjusters to play different roles. AI models have evolved to handle different aspects of claims management, focusing on basic automation, identification and triage functionality. But even with this support, the general setup and roles played by adjusters have stayed the same—AI has simply sped up the process by quickly identifying complex versus simple claims and routing them accordingly.
With the development of GenAI, AI models have moved beyond simple identification to actionable recommendations for different kinds of claims. For simpler claims, AI classifies the claim and recommends fast-tracking to decrease time to completion, enhance customer satisfaction and reduce administrative overhead. Before this development, insurers relied on their own experiences and manual research to decide when a claim can be fast-tracked.
With GenAI, complex claims can be identified quickly and provide well-grounded, precise and data-backed recommendations. This may include suggesting second medical opinions, independent exams and assessments, alternative treatment plans, or even that the insurance provider engages proactively with a claimants’ attorney to review possible early settlement options. Insurance professionals can get detailed explanations from GenAI outputs, which helps them understand exactly what steps to take and when and how to reduce costs and improve recovery outcomes.
GenAI’s Role: Next Best Action in Smart Underwriting
AI in underwriting relies on large datasets to help insurers prepare and price policies that most accurately reflect the underlying risks. These datasets include historical claims, current market trends, and regional risks such as crime rates and environmental hazards, all of which might impact policy costs or outcomes. AI enhances risk assessment by analyzing massive datasets that insurers would never be able to efficiently process manually.
GenAI takes AI-driven underwriting a step further by providing real-time, detailed recommendations and evaluations of risk based on these large datasets. Recommendations are highly contextual and take into account external factors like localized crime rates, environmental hazards, inflation, interest rates, unemployment, regulations and litigation trends.
AI effectively helps workers compensation insurers effectively identify nuances in risk based on the underlying health of the group. For example, if the data shows that three employees have back problems, AI can provide insight and make recommendations into the relative risk of that group for a workers compensation insurer. With this level of insight, underwriters have the knowledge they need to avoid underpricing or overpricing workers comp policies.
GenAI Among the Workforce: Strategies for Effective Change Management
Many insurance professionals fear GenAI’s growth will reduce their roles or replace them in the workforce. This fear has led to a general resistance to AI technology, especially in firms that have not invested in proper AI education or positioning.
For insurance leaders looking to get started with GenAI but who don’t know how to adopt it without inciting panic, here are a few tips for effective change management:
Frame GenAI as a collaborative tool: It’s important to reassure employees that GenAI will not take their jobs or act as a comprehensive insurance solution. Emphasize how it will supplement their valuable knowledge and experience, allowing them to move through the classification of individual claims and policy preparation quickly and more proactively.
Demonstrate GenAI’s value in a concrete way: Actionable insights lead to confident and informed decisions, so make sure the team knows what GenAI can do and what its limitations are. A live demonstration with Q&A is a great way to illustrate the different ways AI can make their jobs easier and less prone to error.
Provide hands-on training and transparent communication: Clear and frequent communication drives adoption and builds trust across all organizational levels. Employees will feel most empowered if they receive hands-on training for how to use these tools for different tasks, as well as any security or compliance procedures they need to follow while using AI.
The Future of GenAI in Insurance
In this new era of GenAI in insurance, underwriters can access detailed AI insights and solid recommendations to better assess risk and rightsize coverage for their clients. On the claims side, insurance professionals can quickly identify optimal settlement paths based on past performance data. This approach supports insurers at all stages of their career, allowing them to make informed decisions in a fraction of the time it would normally take to analyze and derive insights from their data.
As GenAI continues to develop, insurers looking to maximize its impact should prioritize solutions that integrate multi-source insurance data collections to improve data diversity and model training. Expanding beyond a single company’s historical data to include broader industry-wide insights will lead to more robust predictions and recommendations. This approach not only improves operational efficiency but also positions insurers for long-term success as they expand into new verticals and regions.
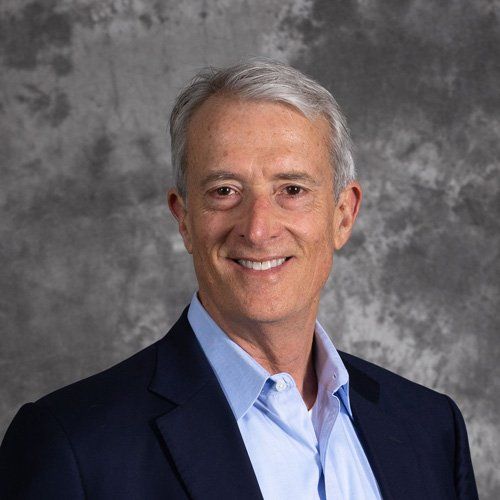
This article first appeared on
Carrier Management.